Addressing Real-World Spatial Problems
Addressing real-world spatial problems is a strategic priority for CSDS for several reasons: 1) To have a positive social impact with our spatial research and tools; 2) to make spatial research and software more accessible to the next generation of high school students who are underrepresented in STEM education; and 3) to allow UChicago students to apply the spatial data analysis skills from class to complex problems that go beyond textbook examples.
This page features examples that illustrate this priority area. Our Assistant Instructional Professors Crystal Bae and Yue Lin are also currently developing capstone projects for students with community partners like Equiticity in Chicago.
STEM Opportunities for Underrepresented Youth
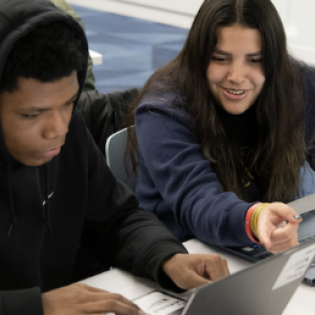
Data4All High School Bridge Program
The bi-annual, 8-week Data4All Bridge workshop connects high schoolers who are underrepresented in STEM to opportunities for learning data science skills not taught in their Chicago Public Schools: Students engage in the thrill of discovery, using Python programming, statistics, and mapping to solve real research problems. Speakers and mentors also introduce them to college and career possibilities in data science that they were not linked to before.
Students learn data science and mapping in small groups with a near-peer mentor (spring 2023)
Data4All is hosted at UChicago’s Data Science Institute (DSI) and was developed in a collaboration between DSI, Argonne National Labs, the Center for Spatial Data Science (Julia Koschinsky is on the curriculum design team), and the Office of Civic Engagement.
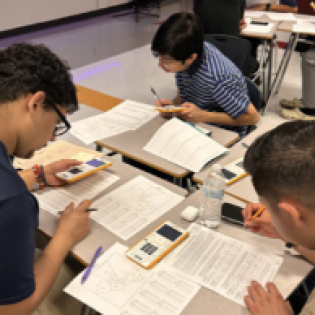
SkewTheScript Sampling Maps
We are a collaborator of SkewTheScript, an innovative new nonprofit to revolutionize how math, statistics and data science are taught in high schools. SkewtheScript makes these STEM subjects relevant to students by focusing on topics students care about, leading to boosts in engagement and achievement. We are helping the nonprofit convert its AP Statistics lesson on sampling methods and income segregation from paper maps for one city to 100 cities, and are creating an interactive web map. In the past two years, SkewtheScript's lessons have been used by over 20,000 teachers and 400,000 students, many from underserved backgrounds.
High schoolers learn about sampling methods based on income & racial segregation in their city
UChicago Coordinator & Mentor: Julia Koschinsky, Center for Spatial Data Science. Mentors: Nico Marchio, Mansueto Institute for Urban Innovation (CSDS Partner) and Dylan Halpern, Open Spatial Lab at Data Science Institute (CSDS Partner; we are using his Nectr web mapping tool). RA: Nikhil Patel (class of '26).
Improved Urban Planning
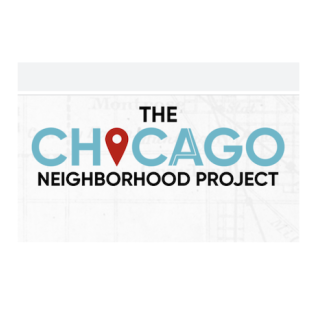
The Chicago Neighborhood Project
The Chicago Neighborhood Project will reveal if current perceptions of neighborhoods are aligned with the historical boundaries currently in use - or, if not, if they should be updated to improve urban planning decisions. Chicago's investments, resident needs assessments, and small business support are often based on boundaries of its 77 community areas - but these are over 100 years old. To assess if these boundaries are outdated, residents are asked to draw their own neighborhood borders in Nov-Dec. 2023.
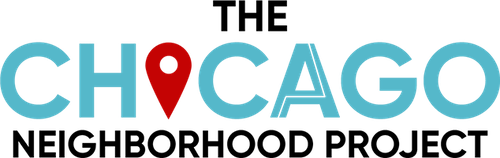
Team: Emily Talen (CSDS Partner: Urbanism Lab), Crystal Bae and Divij Sinha (CSDS), and Lydia Wileden (CSDS Partner: Mansueto Institute for Urban Innovation).
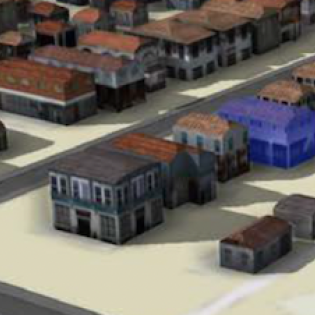
Virtual Reconstruction of Destroyed Black Neighborhoods
Ghost Neighborhoods virtually reconstructs African-American neighborhoods that were destroyed during urban renewal. Led by Ohio State University, with a key technical role by Yue Lin of CSDS, it uses machine learning to provide urban planners, residents, and researchers with 3D map visualizations of what these neighborhoods used to look like. The 3D models will be part of the Poindexter Village Museum and Cultural Center to reconnect with lost assets of black neighborhoods and the stories of residents whose homes were destroyed - and to prevent future urban planning practices from repeating the mistakes of the past.
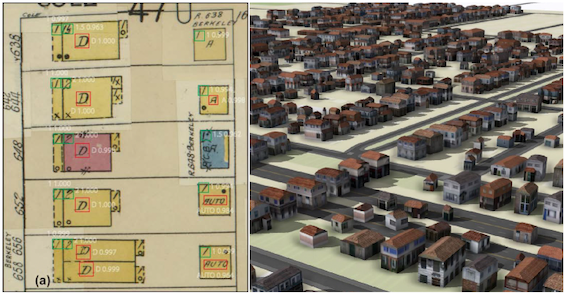
Yue Lin used machine learning to translate historic Sanborn maps (left) to 3D digital models (right)
Fairness in Taxation and Health Services
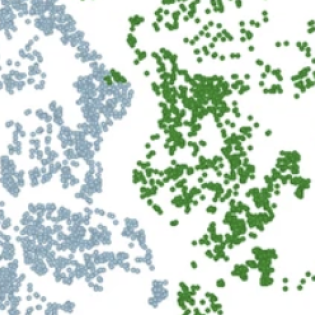
Improving Mass Valuation of Properties
Luc Anselin's and Pedro Amaral's research on endogenous spatial regimes has been used by several local and county governments to improve the estimation of spatial submarkets in the mass valuation of properties. For instance, Pedro Amaral trained staff of the tax assessment office of the City of Belo Horizonte in Brazil in the application of these spatial methods. The methods were used to improve the assessment of property values and derive more accurate estimates of property taxes in this city of more than 2.7 million people.
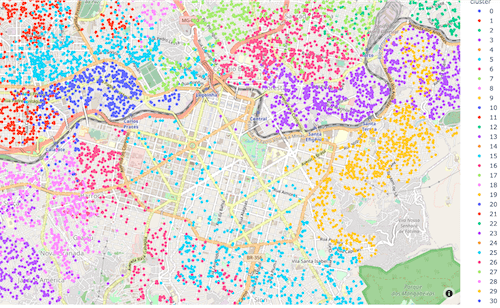
Spatial submarkets in Belo Horizonte's Central Business District detected by the endogenous spatial regimes algorithm
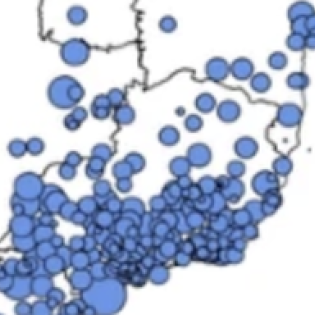
Fair Spatial Provision of Health Equipment
Our spatial research has also supported a more equitable provision of health services. For instance, in another example from Brazil, this research by Pedro Amaral and colleagues identified which areas suffered from gaps in spatial access to key health equipment like dialysis machines, magnetic resonance imaging (MRI), hospital beds, and bone densitometers. The Brazilian Ministry of Health used these results to establish some of the parameters to improve spatial access to this equipment.
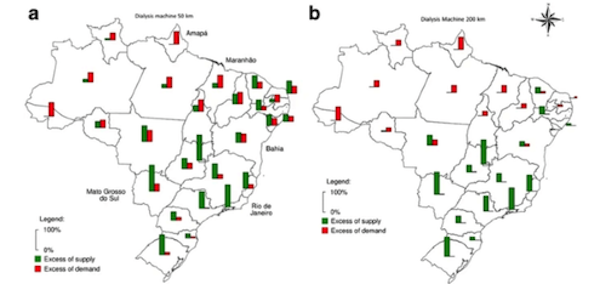
Excess demand (red) vs supply (green) of dialysis machines within 50km (left) and 200km (right)